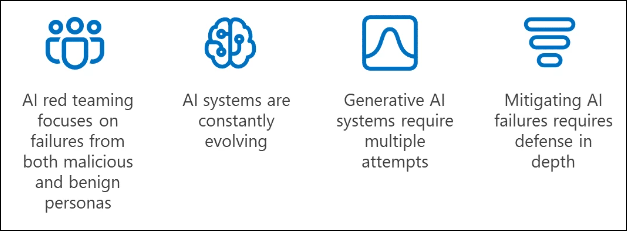
In cybersecurity, “red teaming” refers to the practice of emulating real-world adversaries and their tools, tactics, and procedures to identify risks, uncover blind spots, validate assumptions, and improve the overall security posture of systems.
It can help security teams proactively hunt for failures in AI systems, define a defense-in-depth approach, and create a plan to evolve and grow your security posture as generative AI systems evolve.
Here are some AI red teaming practices suggested by Microsoft Security:
- AI red teaming focuses on failures from both malicious and benign personas
Unlike traditional security red teaming, which mostly focuses on only malicious adversaries, AI red teaming considers broader set of personas and failures. For example, in the new Bing, AI red teaming not only focused on how a malicious adversary can subvert the AI system via security-focused techniques and exploits, but also on how the system can generate problematic and harmful content when regular users interact with the system.
- AI systems are constantly evolving
AI applications routinely change. While traditional software systems also change, AI systems change at a faster rate. Thus, it is important to pursue multiple rounds of red teaming of AI systems and to establish systematic, automated measurement and monitor systems over time.
3. Red teaming generative AI systems requires multiple attempts
Generative AI systems are probabilistic. This means that running the same input twice may provide different outputs. This is by design because the probabilistic nature of generative AI allows for a wider range in creative output. This makes it important to pursue multiple rounds of red teaming in the same operation.
4. Mitigating AI failures requires defense in depth
Just like in traditional security where a problem like phishing requires a variety of technical mitigations such as hardening the host to smartly identifying malicious URIs, fixing failures found via AI red teaming requires a defense-in-depth approach, too. This involves the use of classifiers to flag potentially harmful content to using metaprompt to guide behavior to limiting conversational drift in conversational scenarios.
By following these best practices organizations can effectively identify vulnerabilities and safeguard their technological advancements.